Phoebe Pan, Anusha Srirenganathan Malarvizhi, Chaowei Yang published their research on “Data Augmentation Strategies for Improved PM2.5 Forecasting Using Transformer Architectures”. This study addresses the challenge of predicting extreme air pollution events involving fine particulate matter with diameters less than 2.5 μm (PM2.5), which are linked to an increased risk of cardiovascular and respiratory diseases. Due to the rarity of such events, predictive models often struggle with accuracy. To address this issue, the team employed cluster-based undersampling and Transformer models, experimenting with different cutoff thresholds and partial sampling ratios. The results demonstrated that using a 35.5 μg/m3 cutoff combined with a 20/80 sampling ratio yielded the best performance, particularly for predicting high PM2.5 levels. Models trained on augmented data significantly outperformed those trained on original data, underscoring the effectiveness of resampling techniques. These findings provide valuable insights for improving air quality forecasting, offering benefits for shaping public health and environmental policies aimed at to mitigate the impacts of extreme pollution events such as wildfire, war, and manufacture. This research was funded by NASA AIST and NASA Goddard CISTO membership to the NSF Spatiotemporal I/UCRC (1841520).
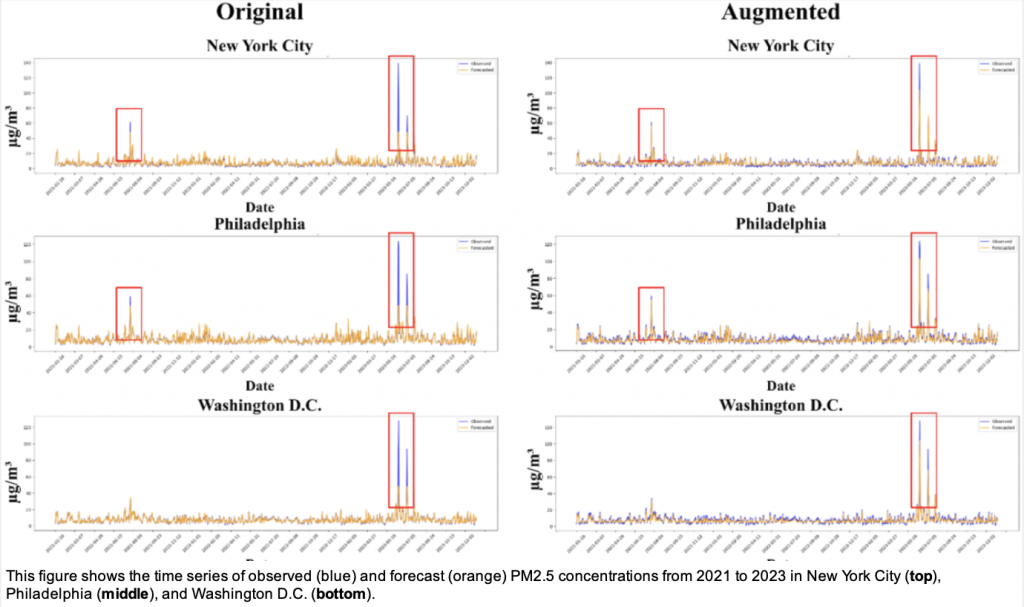